Unlocking New Frontiers with Data Labeling in Machine Learning
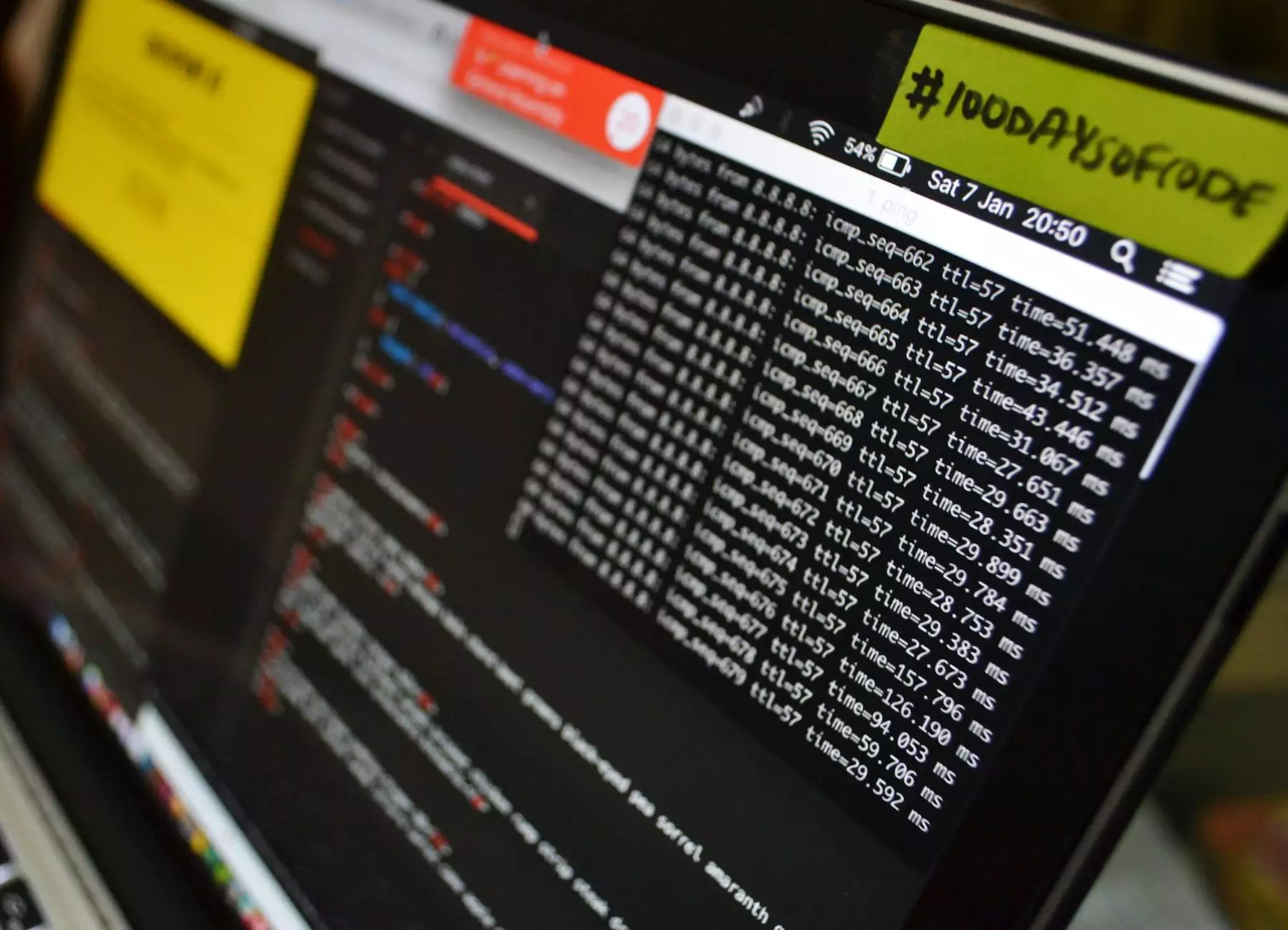
The Vital Role of Data Labeling in Machine Learning
In the realm of machine learning, the significance of data labeling cannot be overstated. As technology evolves, the demand for accurately labeled data continues to surge, serving as the backbone of successful AI applications. Data labeling involves the meticulous process of assigning informative labels to data inputs, ensuring machines can interpret and act upon them effectively.
Every AI model's performance hinges on the quality of its training data. The process of accurately labeling this data is crucial for enabling machine learning algorithms to recognize complex patterns, make predictions, and ultimately deliver valuable insights across various industries.
Understanding the Data Annotation Process
The data annotation process typically involves several stages, each integral to the overall quality of the labeled dataset. Here’s a brief overview:
- Data Collection: Gathering raw data from various sources, such as images, videos, text, or audio.
- Data Preparation: Cleaning and organizing the data to ensure it's suitable for labeling.
- Annotation: Assigning labels to data points using specialized tools and platforms.
- Quality Assurance: Reviewing labels for accuracy and consistency, often through multiple rounds of validation.
- Deployment: Utilizing the labeled dataset to train machine learning models for real-world applications.
Types of Data Annotation Tools
Businesses like keylabs.ai offer a variety of data annotation tools tailored to meet specific requirements. Here are some common types:
- Image Annotation Tools: Used for labeling images with bounding boxes, segmentation masks, or key points, facilitating the training of computer vision models.
- Text Annotation Tools: Essential for labeling text data, from identifying entities in natural language processing (NLP) to sentiment analysis.
- Audio Annotation Tools: Designed for transcribing spoken language, identifying speakers, and other tasks crucial for speech recognition applications.
- Video Annotation Tools: Allow for frame-by-frame labeling to detect objects, actions, and events in video data.
The Importance of Choosing the Right Annotation Platform
Selecting the right data annotation platform is critical in ensuring that your data labeling machine learning efforts yield high-quality, reliable results. Various factors should be considered when choosing a platform, including:
- Scalability: The platform should be capable of handling increasing volumes of data as your project grows.
- Accuracy: Look for platforms that prioritize human verification processes, enhancing label accuracy.
- Integration Capabilities: A good platform should seamlessly integrate with your existing data infrastructure.
- User-Friendly Interface: To ensure efficiency, the platform should be easy to navigate for your team.
- Cost-Effectiveness: Evaluate the pricing structure and overall value based on your project’s budget.
Benefits of Data Labeling in Machine Learning
The benefits of effective data labeling for machine learning applications are extensive and transformative:
- Improved Model Accuracy: Well-labeled data directly correlates with model performance, allowing algorithms to learn better.
- Enhanced Predictive Insights: Accurate annotation equips models to provide more reliable predictions in various applications, from finance to healthcare.
- Faster Development Cycles: Automated labeling tools can speed up the process, allowing teams to iterate and refine models rapidly.
- Cost Savings: Investing in effective labeling upfront can minimize errors and reduce the costs associated with retraining models later.
- Better Understanding of Data: The labeling process encourages teams to engage deeply with their data, fostering insights that can inform business decisions.
Challenges in Data Labeling
Despite its benefits, data labeling comes with its own set of challenges:
- Time-Consuming: Manual labeling of large datasets can be labor-intensive and demoralizing for teams.
- Subjectivity: Different annotators might interpret labeling guidelines differently, leading to inconsistencies.
- Scaling Issues: As data volumes grow, maintaining quality while increasing throughput becomes a significant challenge.
- Resource Allocation: Finding skilled annotators and managing resources efficiently can be a daunting task.
Automating the Data Labeling Process
To mitigate some of the challenges associated with manual data labeling, many organizations are turning to automation. Automated annotation tools utilize machine learning algorithms to streamline the labeling process, reducing the burden on human annotators. These tools can significantly enhance productivity and allow teams to focus on more nuanced tasks that require human intelligence.
However, while automation can add value, it’s essential to maintain a balance between human and machine inputs. Human oversight ensures that the quality remains high, thus leveraging both the speed of automation and the accuracy of human judgment.
How keylabs.ai Enhances Your Data Annotation Strategy
Keylabs.ai stands at the forefront of data annotation platforms, offering robust solutions that cater to businesses looking to excel in machine learning applications. Here’s how keylabs.ai can elevate your data annotation strategy:
- Comprehensive Annotation Features: Our platform supports various data types, including images, text, and audio, enabling a holistic approach to data labeling.
- High-Quality Outcomes: Rigorous quality assurance processes ensure that every labeled dataset meets the highest standards.
- Multi-Language Support: Expand your reach by accessing tools that accommodate different languages and locales.
- User-Friendly Interface: The intuitive design allows teams to label datasets efficiently and effectively.
- Scalable Solutions: Our platform can grow with your business, making it suitable for both small startups and large enterprises.
By choosing keylabs.ai, you position your organization to harness the full potential of data labeling machine learning, ultimately driving your AI initiatives forward.
Conclusion: Embracing the Future of Data Annotation
As we stand on the brink of advancements in artificial intelligence, the role of data labeling in machine learning has never been clearer. Accurate, efficient, and well-managed labeling processes are foundational to the development of effective AI applications. By leveraging leading platforms like keylabs.ai, businesses can harness quality data annotation to unlock new insights, enhance their models, and stay ahead of the competition. The future of machine learning is bright, driven by the power of data, and it all begins with meticulous data labeling.